Are you aspiring to become a Data Scientist or Machine Learning expert? You must be worried about the job market in this field, right?
According to Click Digital, the job market for Machine Learning/Data Science is undergoing significant changes. So what’s the real situation, is this field still as hot as before? Let’s delve into it with Click Digital and find some useful tips for you!
Table of Contents
The Reality of ML/DS Jobs: Has the Fever Broken?
You might notice that finding jobs in Machine Learning/Data Science in Australia and Vietnam is currently facing some challenges. People often mention big companies like AWS, Atlassian, or TikTok as “golden doors” for young talents. However, the reality is that these companies primarily recruit mid to senior-level personnel.
Most small and medium-sized companies either don’t hire or have extremely high requirements, making it almost impossible for fresh graduates to meet their standards. This situation makes it difficult for many new graduates to find jobs in this field.
Is it really a shortage of jobs in this field, or are you just not searching in the right places?
The State of ML/DS Jobs: Time to Be Realistic!
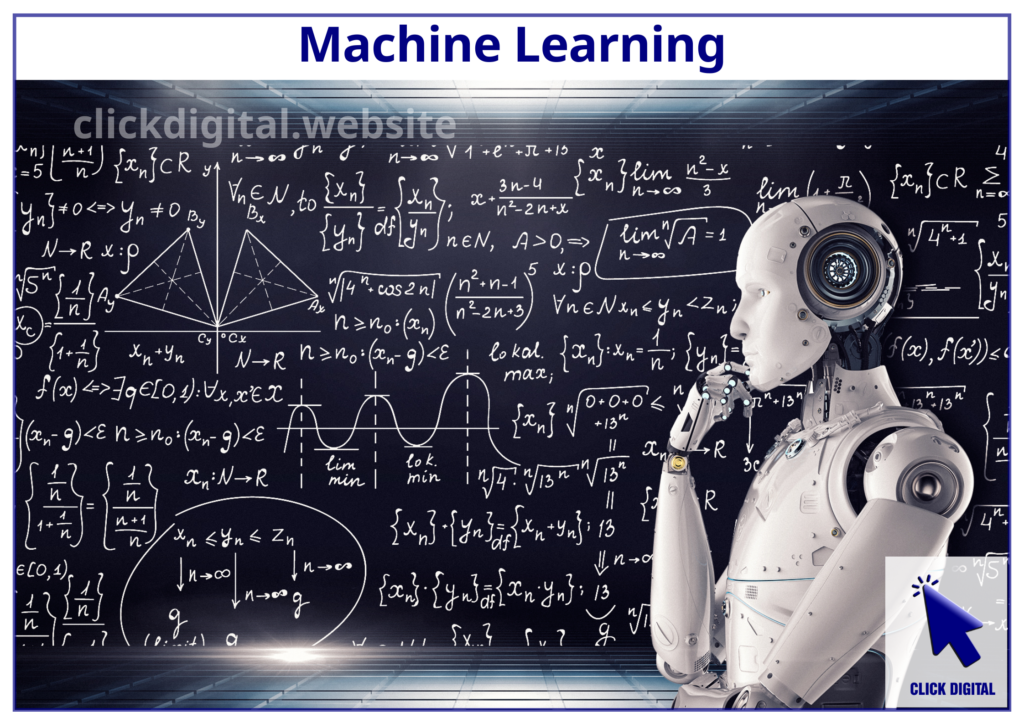
The harsh reality is that the current market demands individuals with practical experience, capable of solving problems and delivering value to businesses.
Here are some reasons why new graduates struggle to find jobs:
- Lack of practical experience: Companies seek individuals who can work immediately and generate profits, not those who require extensive training.
- High demands: Companies need highly skilled individuals who can solve complex problems.
- Intense competition: The number of people studying this field is constantly increasing, leading to fierce competition in the job market.
You might wonder: Should I pursue a Master’s degree?
Pursuing a Master’s degree is not always the best solution. Many universities treat education as a business and prioritize profit over ensuring quality education and employability after graduation. Moreover, a Master’s degree doesn’t automatically guarantee a job. In fact, the ML/DS job market in Europe is also very competitive, requiring a higher degree like a Master’s or PhD, along with practical experience.
Comparison of the Advantages and Disadvantages of Pursuing a Master’s Degree vs. Gaining Practical Experience:
Factor | Pursuing a Master’s Degree | Gaining Practical Experience |
Advantages | – Enhances specialized knowledge – Expands network – Increases competitiveness | – Has practical experience – Understands market demands – Enters the profession quickly |
Disadvantages | – High costs – Long study duration – No guarantee of finding a job – Might not align with market demands | – Low income – May face difficulties in finding opportunities – Expertise might be limited |
Suitable for | – Those who want in-depth research – Those who want to pursue an academic career – Those with good financial resources | – Those who want to quickly enter the profession – Those who enjoy learning and working practically – Those who can self-learn and adapt quickly |
Change your strategy!
Instead of pursuing further education, focus on gaining practical experience to enhance your value. Consider taking on real projects (even unpaid ones, as they often offer more practical experience than a Master’s degree).
Tips for you: How to increase your chances of finding a job
- Unpaid work: Look for unpaid opportunities at companies, even if it involves tasks like data labeling, HTML/CSS coding, etc.
- Learn practical knowledge: Focus on practical knowledge that companies need, such as React, Kafka, etc.
- Build a Portfolio: Create a portfolio showcasing your real-world projects. This could include personal projects, participation in coding competitions, etc.
- Join communities: Join Machine Learning/Data Science communities to gain experience and connect with experienced individuals.
Additionally:
- Research the job market thoroughly: Before deciding on a field, thoroughly research the job market and the necessary skills.
- Be proactive: Actively seek job opportunities, attend conferences, and connect with people in the industry.
- Don’t give up: The job market is always competitive, but with sufficient knowledge, skills, and perseverance, you will succeed.
You might consider pursuing Data Engineering if you’re struggling to find jobs in ML/DS. Data Engineering is a related field with more opportunities.
To find a job in ML/DS, you need basic programming skills, knowledge of Machine Learning and Deep Learning, and problem-solving abilities.
Analysis of Factors Affecting Job Search in ML/DS
Factor | Impact | Solutions |
Practical experience | Crucial. Companies prioritize individuals who can solve real-world problems and create value immediately. | Unpaid work, personal projects, internships, volunteer work, etc. |
Specialized skills | Need to have a strong understanding of Machine Learning, Deep Learning, data analysis, natural language processing, etc. | Learn through online courses, books, join communities, practice with personal projects, etc. |
Programming skills | Mastery of at least one popular programming language in the ML/DS field (Python, R, Java, etc.) is essential. | Learn programming, practice coding regularly, participate in coding competitions, etc. |
Portfolio | Shows your skills and practical experience through completed projects. | Build a portfolio with personal projects, internship projects, volunteer projects, etc. |
Network | Helps you connect with industry professionals, find job opportunities, and gain experience. | Join ML/DS communities, connect with industry professionals through conferences, events, etc. |
Competition | The ML/DS market is highly competitive. | Enhance your skills, build an impressive portfolio, network, proactively search for opportunities, etc. |
Job market | The demand for ML/DS talent is changing, with large companies often hiring senior-level individuals. | Thoroughly research the market, understand hiring needs, choose a suitable profession, etc. |
Adaptability | The ML/DS field is constantly evolving, requiring a willingness to learn and adapt quickly. | Always update your knowledge, follow new trends, self-learn, etc. |
Comparison of the Advantages and Disadvantages of Pursuing ML/DS vs. Data Engineering:
Factor | Machine Learning/Data Science | Data Engineer |
Specialization | Focuses on building and training ML/DL models to solve complex problems | Builds and manages data systems, ensuring efficient data storage, processing, and retrieval |
Skills | Data analysis, model building, model training, model testing and evaluation, natural language processing, computer science | Programming, database management, data architecture, system design, big data processing, computer science |
Job opportunities | High competition, mainly at large companies, requires experience and advanced skills | Diverse job opportunities, both at large and small companies, can pursue various career paths |
Salary | Often higher than Data Engineers | Often lower than ML/DS, but still competitive |
Challenges | Requires continuous knowledge updates, using new technologies, solving complex problems | Managing large datasets, data security, building efficient systems, updating new technologies |
Note: This table is for reference only. Each individual should choose a profession that suits them based on their interests, skills, and career goals.
Conclusion: The Key to Success in ML/DS
- Focus on learning and refining practical skills: Work on personal projects, participate in competitions, etc., to enhance your abilities.
- Build a network: Connect with people in the industry to gain experience and explore job opportunities.
- Stay updated: The Machine Learning/Data Science field is rapidly evolving, so keep your knowledge up-to-date to stay ahead of the curve.
- Learn to present yourself effectively: Learn to write a resume, participate in interviews effectively, and showcase your skills professionally.
Remember, success in Machine Learning and Data Science is a long journey. There are no shortcuts!
Note: This article is based on personal opinions and experiences. Everyone may have different perspectives.